How You Split Matters: Data Leakage and Subject Characteristics Studies in Longitudinal Brain MRI Analysis
Published in MICCAI FAIMI, 2023
Dewinda Julianensi Rumala
Publication Preprint Code Poster Video
This work has been honored with the Best Poster Presentation Award at MICCAI FAIMI 2023 workshop.
Download Poster View Award Certificate
Introduction
Neglect of Longitudinal Data: In medical image analysis, the significance of longitudinal data is often overlooked. Longitudinal data, with its repeated observations over time, holds a wealth of information critical for understanding changes and patterns over the progression of diseases, offering insights into treatment efficacy.
Data Handling in Longitudinal Analysis: Proper handling of longitudinal data is a crucial aspect that often receives inadequate attention. Inconsistent or improper data handling procedures can introduce biases and compromise the robustness and reliability of the results, even in 3D-based medical image analysis.
Deep Learning Performance Challenges: Deep learning models, despite their high performance, can be misleading in the medical imaging domain. Biases, such as data leakage, can give overly optimistic results during evaluation, potentially leading to incorrect conclusions. Understanding and mitigating these challenges are vital for trustworthy AI-powered medical diagnoses.
Methods
- Deep Learning model: 3D CNN (DenseNet121)
- Datasets: 3D Longitudinal T1-weighted and T2-weighted MRI from ADNI (Dataset statistics see Table S1)
- Data Processing: CAT12 with VBM pipeline
- Purpose: Three-way classification (CN, MCI, and AD)
Table S1. Dataset Statistics. The ratio of females to males and the average age are based on the number of images rather than the number of subjects.
Collection | Data Group | No of Subjects | Female / Male | Age | No of Scans | |
Before Augmentation | After Augmentation | |||||
5-Fold data | CN | 41 | 85/65 | 76.68 ± 4.15 | 150 | 300 |
MCI | 45 | 50/100 | 74.19 ± 8.57 | 150 | 300 | |
AD | 25 | 30/20 | 74.22 ± 8.90 | 50 | 300 | |
Hold-out data | CN | 8 | 17/13 | 74.81 ± 3.13 | 30 | - |
MCI | 11 | 8/22 | 75.42 ± 7.26 | 30 | - | |
AD | 7 | 16/1 | 78.26 ± 6.52 | 30 | - |
Evaluation Scheme
Data Splitting Strategies during CV:
- Subject-wise Splitting
- Record-wise Splitting
- Late Splitting
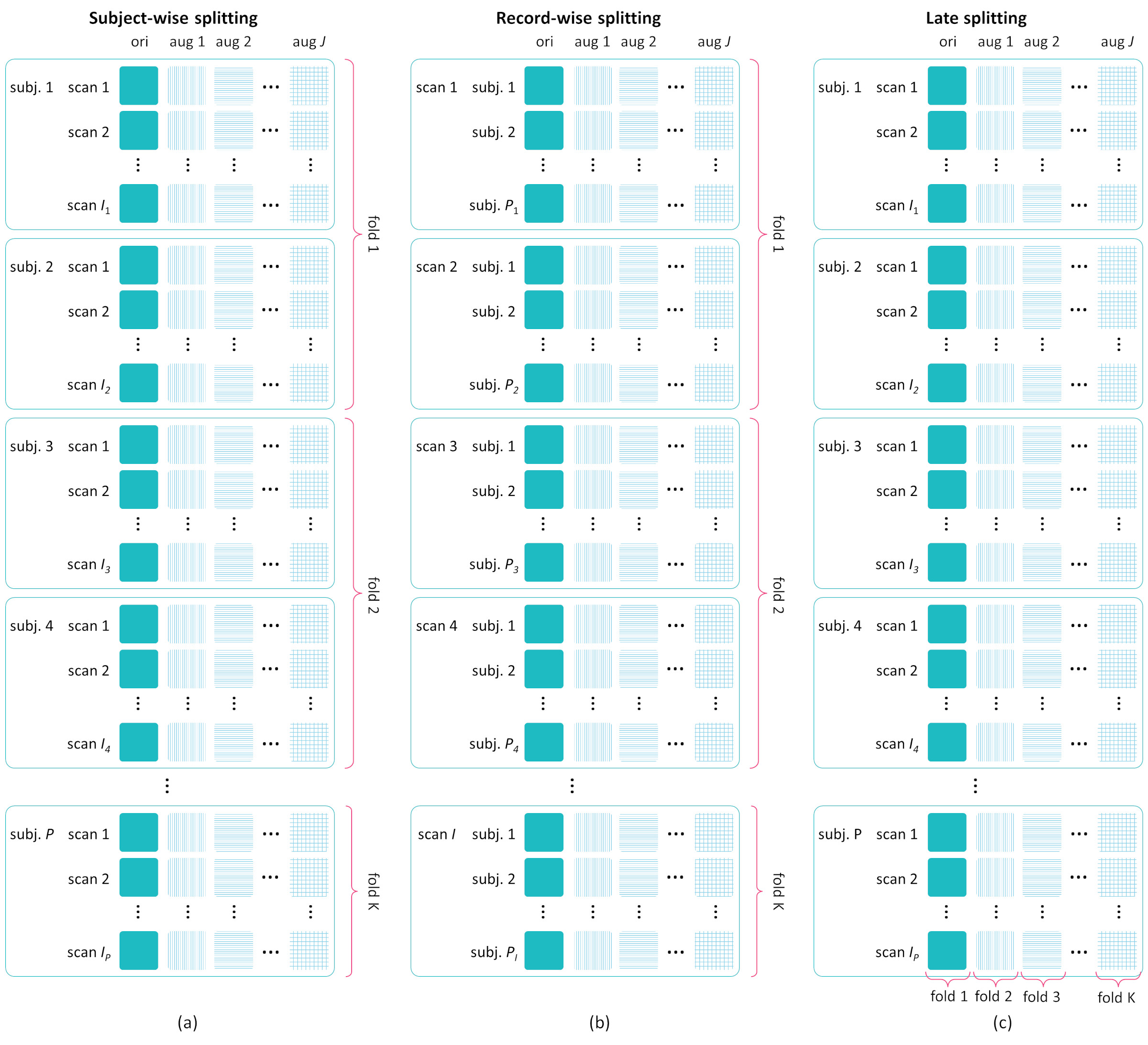
Training Setups
Table S2. Overview of the parameters used across all experiments: the learning rate was reduced using the ReduceLROnPlateau scheduler from TensorFlow by a factor of 0.1 when validation loss did not decrease after 10 epochs. Adam was used as the optimizer.
Parameter | Value |
---|---|
Learning rate | 0.0001 |
Epsilon | 0.0001 |
Beta 1 | 0.9 |
Beta 2 | 0.99 |
Epoch | 100 |
Batch size | 24 |
Results and Analysis
- Data Splitting Strategy Impact:
- Data splitting strategy significantly influences model performance (P=0.0389).
- Record-wise split excels during CV, closely followed by late split.
- Surprisingly, subject-wise split performs poorest during CV but generalizes best to hold-out data.
- MRI Sequence Influence:
- Choice between T1 and T2 MRI sequences does not significantly affect overall classification performance (P=0.7921).
- Both sequences (T1 and T2) prove suitable for the three-way classification task of CN, MCI, and AD.
- Insights from GradCAM Visualization:
- Presence of shortcut learning found frem certain data splitting strategies: record-wise and late splits.
GradCAM Visualization
Gif Samples
Data Group | Subject-wise Split | Record-wise Split | Late Split |
CN | ![]() [O] Correctly classified | ![]() [O] Correctly classified | ![]() [O] Correctly classified |
![]() [X] Misclassified | ![]() [X] Misclassified | ![]() [X] Misclassified | |
AD | ![]() [O] Correctly classified | ![]() [O] Correctly classified | ![]() [O] Correctly classified |
![]() [X] Misclassified | ![]() [X] Misclassified | ![]() [X] Misclassified |
Discussion
- How You Split Matters
- The choice of data splitting strategy during cross-validation significantly influences the performance and robustness of deep learning models in longitudinal medical image analysis.
- Data Leakage and Identity Confounding
- Improper data splitting can lead to data leakage, causing identity confounding within the models. This compromises their generalization and can result in misleadingly optimistic performance assessments.
- Shortcut Learning Revealed by GradCAM
- Visualization using GradCAM highlights potential shortcut learning in models, particularly from record-wise and late-wise splitting strategies. This suggests that models may learn unintended patterns during cross-validation.
- Validating Robustness with Subject-Wise Split
- This study validates previous findings suggesting a promising approach—subject-wise split demonstrates relative robustness and less vulnerability to data leakage compared to record-wise and late splitting strategies. However, challenges like underperformance indicate the need for further investigation, potentially with a larger and more diverse dataset.
- Future Directions
- Promoting Subject-wise split: future research should strongly consider subject-wise split for more reliable model evaluation and development.
- Investigating data variance and sensitive attributes: Further research should delve into the correlation between data splitting strategies and data variance, particularly exploring the influence of sensitive attributes such as age and sex. Understanding these relationships can lead to more nuanced and fair models.
Limitations
- Generalization Challenges:
- Limited generalization to hold-out data likely influenced by data variance in sensitive attributes (e.g., age and gender imbalance).
- Robustness of Subject-Wise Split:
- Despite a significant drop in performance, subject-wise splitting demonstrates relative robustness. Addressing potential underfitting by incorporating a more diverse subject pool could enhance this approach’s performance. Subject-wise splitting strategy shows more robustness but still suffers from performance drops, possibly due to underfitting, which can be mitigated with a larger and more diverse dataset.
References
- Chaibub Neto, E., Pratap, A., Perumal, T.M., Tummalacherla, M., Snyder, P., Bot, B.M., Trister, A.D., Friend, S.H., Mangravite, L., Omberg, L.: Detecting the impact of subject characteristics on machine learning-based diagnostic applications. npj Digital Medicine 2(1), 99 (Oct 2019). https://doi.org/10.1038/s41746-019-0178-x
- Yagis, E., Atnafu, S.W., García Seco de Herrera, A., Marzi, C., Scheda, R., Giannelli, M., Tessa, C., Citi, L., Diciotti, S.: Effect of data leakage in brain MRI classification using 2D convolutional neural networks. Scientific Reports 11(1), 22544 (Nov 2021). https://doi.org/10.1038/s41598-021-01681-w
Acknowledgement
Special thanks to Directorate General of Higher Education and Research Technology, Indonesia, Prof. I Ketut Eddy Purnama (Sepuluh Nopember Institute of Technology, Indonesia) and Prof. Tae-Seong Kim (Kyung Hee University, South Korea).